10 Uncommonly Useful Observations on Product-Market Fit for Data Products
Forget what you know about product-market fit for traditional SaaS—data products play by a different rulebook. In this post, I share ten uncommonly useful observations from my years in the healthcare data trenches, challenging conventional wisdom and providing a roadmap for success in the complex wo
As a veteran in the healthcare data product space, I've seen my fair share of successes and failures when it comes to achieving product-market fit. While many principles from traditional SaaS products apply, data products have their own unique challenges and opportunities. Here are ten uncommonly useful observations that can help you navigate the complex landscape of product-market fit for data products.
1. The "Aha!" moment is often delayed
Standard SaaS expectation: Users should have an immediate "Aha!" moment upon first use.
Data product reality: The true value of a data product often emerges over time as patterns and insights accumulate. Your onboarding process needs to set the right expectations and provide early wins while building towards the bigger picture.
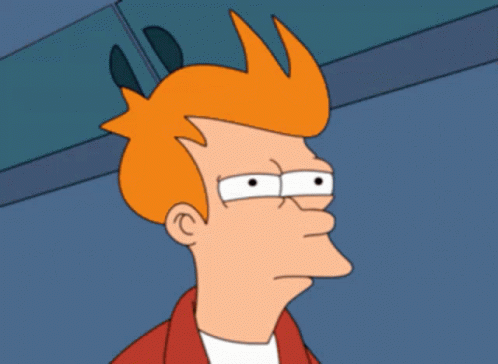
2. Your product is only as good as your data sources
Standard SaaS expectation: Product quality is primarily determined by features and user experience.
Data product reality: The quality, freshness, and relevance of your data sources can make or break your product. Invest heavily in data acquisition, cleansing, and integration. Remember, garbage in, garbage out.
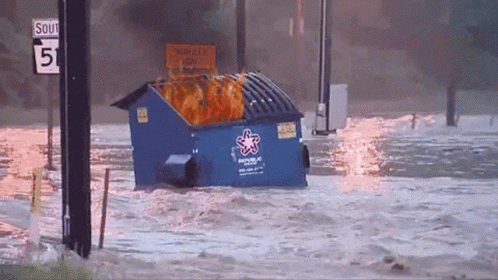
3. Customization is not just a feature, it's a necessity
Standard SaaS expectation: One-size-fits-all solutions with minor customization options.
Data product reality: Every organization's data landscape is unique. Your product needs to be flexible enough to accommodate diverse data structures, integration points, and use cases without becoming overly complex.
4. The sales cycle involves education and change management
Standard SaaS expectation: Demonstrate value quickly and close the deal.
Data product reality: Selling a data product often requires educating prospects on data literacy, changing existing processes, and aligning multiple stakeholders. Your sales process should include elements of consultative selling and change management.
5. Success metrics are often indirect
Standard SaaS expectation: Direct metrics like user engagement and feature adoption indicate success.
Data product reality: The true impact of your data product might be several steps removed from direct usage. Success could manifest as better decision-making, cost savings, or revenue growth for your clients. Develop ways to track and attribute these indirect benefits.
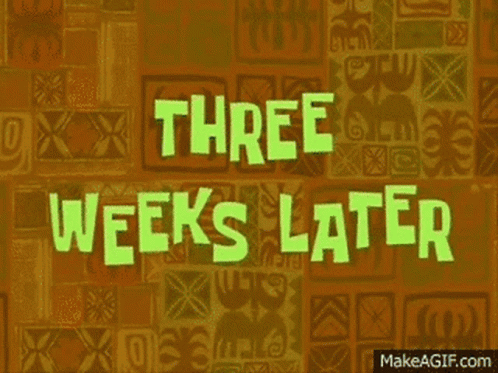
6. The "network effect" is data-driven
Standard SaaS expectation: More users lead to more value through increased interactions.
Data product reality: More data often leads to better insights and predictions. Consider how you can create virtuous cycles where using your product generates more valuable data, which in turn makes the product more valuable for all users.
7. Regulatory compliance is a feature, not a bug
Standard SaaS expectation: Compliance is a necessary evil.
Data product reality: In regulated industries like healthcare, robust compliance features can be a major selling point. Embrace compliance as a core feature and competitive advantage, not just a checkbox.
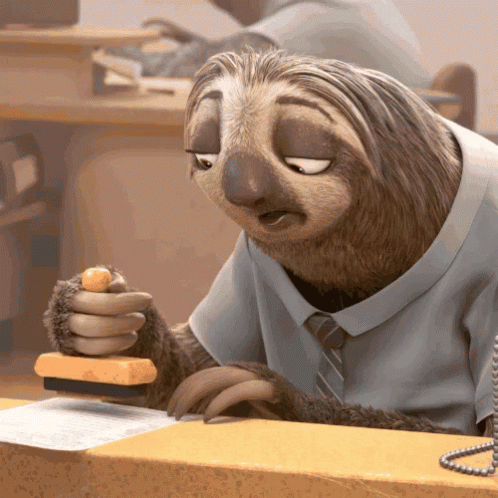
8. The product evolves with the data science field
Standard SaaS expectation: Periodic feature updates based on user feedback and market trends.
Data product reality: Advances in data science and machine learning can fundamentally change what's possible with your product. Stay on the cutting edge and be prepared to make significant pivots as new techniques emerge.
9. User personas include both humans and algorithms
Standard SaaS expectation: Focus primarily on human end-users.
Data product reality: Your product might need to serve both human analysts and automated systems or AI models. Design your APIs and data outputs with both in mind.
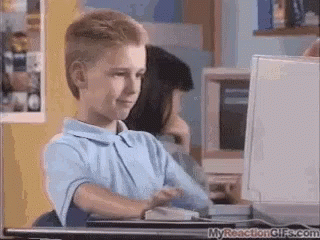
10. The MVP is more complex but potentially more powerful
Standard SaaS expectation: Build a simple MVP to test core assumptions quickly.
Data product reality: Your MVP needs to include not just basic features, but also data pipelines, quality checks, and initial models or analyses. While this makes the MVP more complex, it also means you're testing a more complete value proposition from the start.
Conclusion
Achieving product-market fit for data products requires a nuanced understanding of the unique challenges and opportunities in this space. By keeping these observations in mind, you can avoid common pitfalls and focus on the elements that truly drive value for your users.
Remember, in the world of data products, your goal isn't just to fit the market — it's to evolve with it, shape it, and ultimately, to help your users make better decisions through the power of data.