General Product Management vs Data Product Management: Let's Get Specific
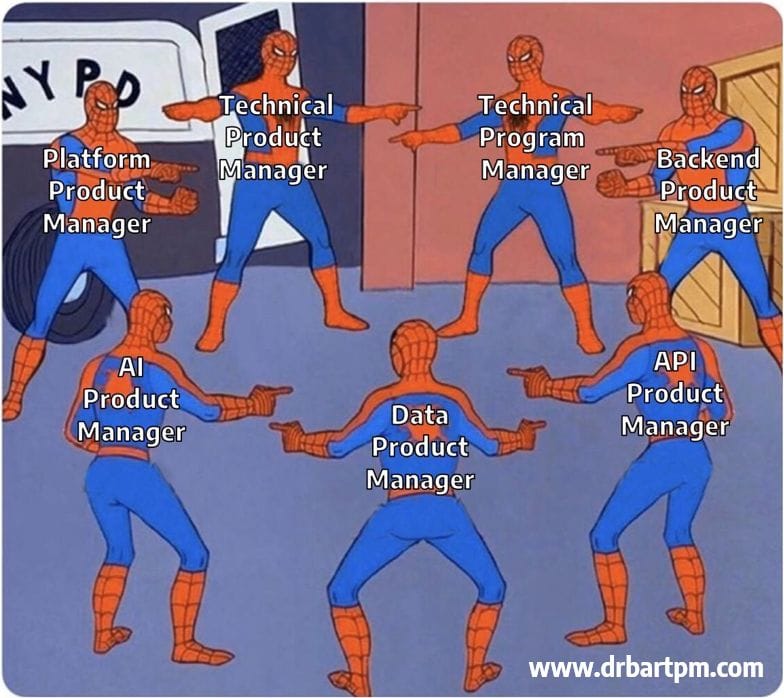
Growth is driven by compounding, which always takes time. Destruction is driven by single points of failure, which can happen in seconds, and loss of confidence, which can happen in an instant. - Morgan Housel, Psychology of Money
Working with data requires holding two things true at once. You must constantly investigate, experiment, and explore while building certainty, understanding, and stability. It’s about trust. Bringing some order to chaos which is what data is.
General product management - a vague notion I know but think of any physical product or technical service - relies on the former truth with the latter coming as the investigation comes to a close.
When building with data, there is always something new with your input. The ground is shifting under your feet. It makes sense to flow with it.
Over the years of building data products and teams, I’ve found a couple of differences. Not massive but real. And worth talking about.
Core Focus & Responsibilities
- User-Centric DNA: Everything revolves around user needs and experiences
- Broad Scope: Lives at the intersection of engineering, business, and UX
- Qualitative Decision-Making: Heavy reliance on user feedback and market insights
Data Product Management:
- Data-Centric DNA: Data shapes every decision and feature
- Specialized Scope: Operates where engineering, business, and data meet
- Quantitative Decision-Making: Lives and dies by metrics and experiments
This is a dramatic simplification. A data product manager is a product manager first. Jason Cohen writes, “the role centers on deciding what to build.” It’s deceptively simple and a data product manager has to do the same. The difference lies in the material and constraints.
A SaaS PM is product focused and while they have to manage the business viability risk (can the business support and benefit from this product), they often have fewer hard constraints on what to build with.
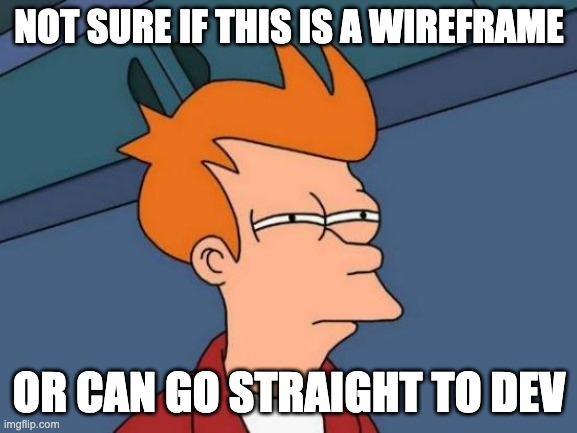
The Skills Gap That Actually Matters
General PMs need:
- Market research chops
- Customer development expertise
- Business strategy understanding
- Basic technical knowledge
In addition to those core things, Data PMs require:
- Reasonable data science understanding
- Machine learning/AI experience
- Data governance appreciation
- Analytics proficiency
This is asking a lot of one person. True. Add in some element of data expertise and you have a future founder on your hands.
The demands are impossible. The job is technically impossible. That's why you are on a team. It's not on you but you need to bring context, creativity, and an ability to coach.
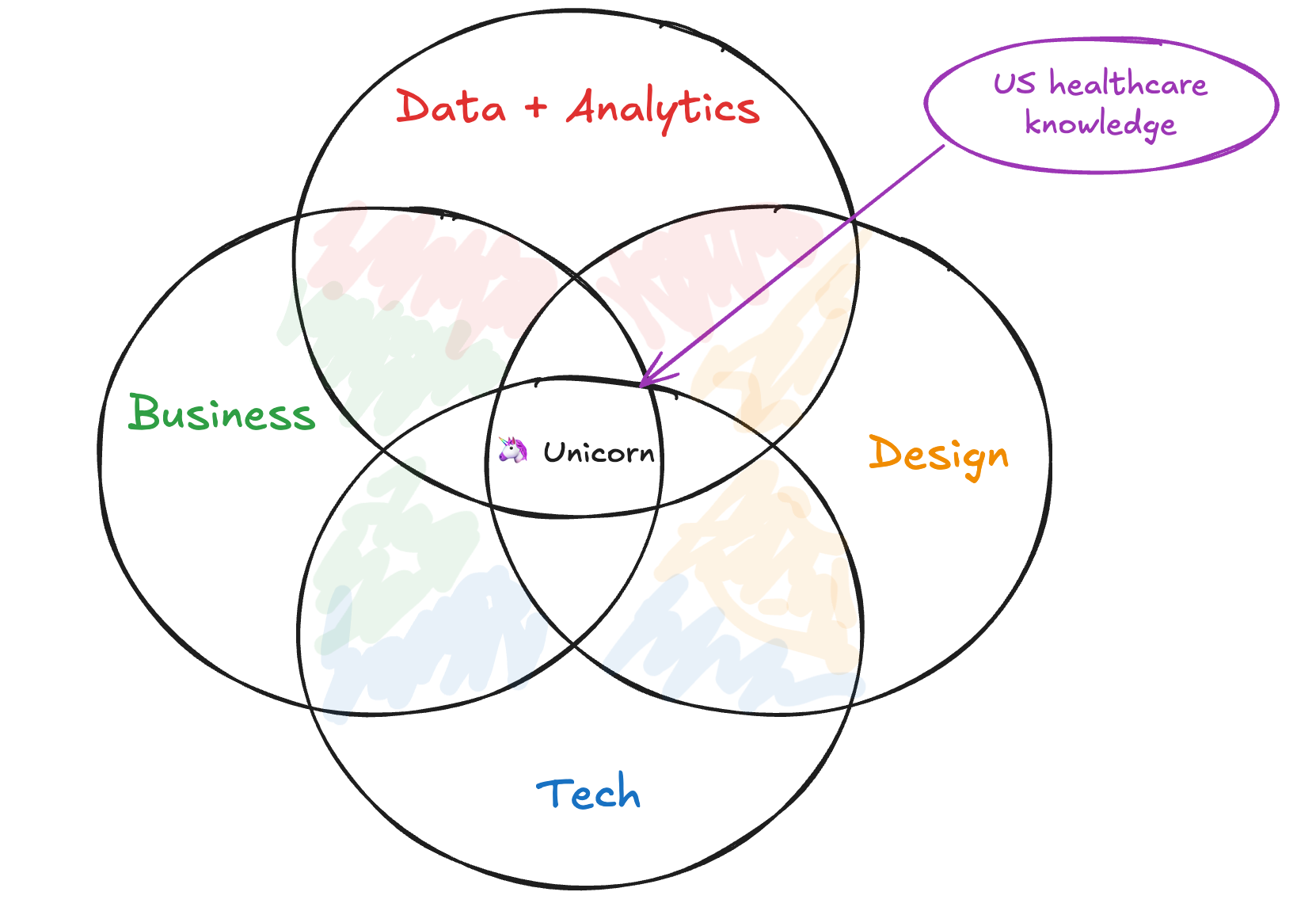
Team Dynamics & Collaboration
General PM Teams often include:
- Cross-functional squads (engineers, designers, marketers) helmed by the Product Trio (PM, Tech Lead, Design Lead)
- Customer feedback drives development
- Focus on feature shipping and user adoption
Data PM Teams:
- A similarly cross-functional squad but helmed by a Product Quad (Data PM, Tech Lead, Design Lead, and Data Lead) with data-centric specialists (data scientists, data engineers, analysts) added in
- Data quality drives development
- Focus on data accessibility and democratization
Data products are inherently more complex. Especially if they are B2B which 90% of them are. Balancing the quality risk along with the other four (value, usability, feasibility, and business viability per Marty Cagan) is just icing on the cake.
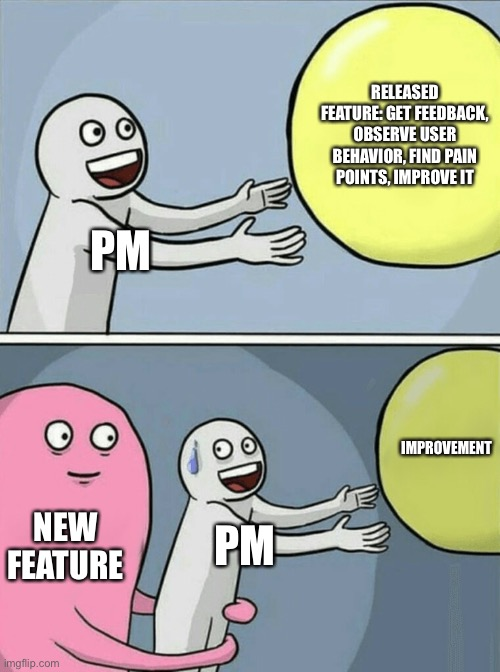
The Healthcare Reality Check
In healthcare, these differences become even more pronounced. When I was leading data product at Revive, we weren't just shipping features - we were shipping trust. We had to build the story for our healthcare partners while still maintaining strict controls on protecting patient data.
Healthcare B2B is "playing on hard mode". Building a data product in a regulated industry like US healthcare requires a focus on quality risk above all else.
Four Key Shifts That Nobody Talks About:
- The Risk Profile
- General PM: Ship (potentially) buggy code, push a fix
- Data PM: Ship bad data, lose trust forever
- The Success Metrics
- General PM: Usage, engagement, retention
- Data PM: Data quality, decision velocity, trust signals
- The Customer Journey
- General PM: "Wow, this is great!"
- Data PM: "I don't trust this yet... but maybe..."
- The Team Dynamic
- General PM: "When can we ship?"
- Data PM: "How do we validate?"
Looking Ahead
As AI and ML become ubiquitous, these roles will continue to merge. But the fundamental differences in approach - particularly around risk, trust, and validation - will remain critical. As noted in the lead quote, growth is compounding, in markets and products, but trust can be lost in an instant.
Every product will have data components. But not every product will be a data product. Understanding this distinction will be an important skill for the next generation of PMs.
The Questions Nobody's Asking
- How do we measure trust as a product metric?
- What does "move fast and break things" look like when data quality is non-negotiable?
- How do we build data products that balance innovation with reliability + trust?
Would love to hear your thoughts. What differences have you noticed between Data PM and General PM roles in your work?