The Rise of Data Product Managers (DPMs) in the Age of Generative AI
In this era of frothy "generative AI" talk and valuations, data has become the lifeblood of organizations, powering innovation and fueling competitive advantage. The much-hyped AI models and products are so fascinating because they were trained on vast amounts of curated, labeled, and sourced data - both clean and messy - collected over the past few decades.
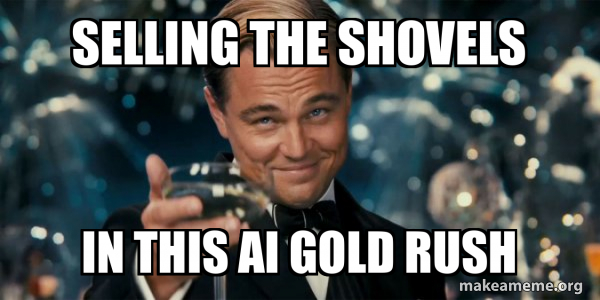
Yet, amidst the rush to invest in sophisticated data infrastructures like warehouses and lakes, a critical disconnect has emerged. Executives often find themselves in the dark when it comes to harnessing the true value of their data assets. It's like they're sitting on a treasure trove but haven't quite figured out how to unlock it. As a result, data teams are undervalued, their challenges unaddressed, and their potential constrained by inadequate resources.
We have powerful hammers in our hands, trying to find those nails. If the aim (clean data and understanding) is only slightly off, self-inflicted damage is highly likely.
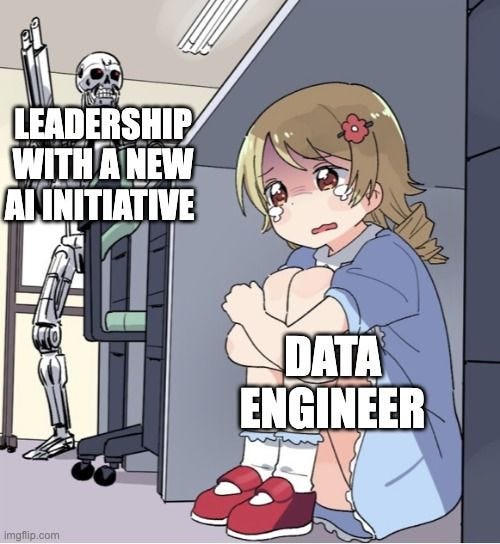
Enter the Data Product Manager (DPM) – the unsung hero who's about to shake things up and revolutionize the way we leverage data in the age of AI. A continuation of the BI trend and analytics in organizations.
The ideal DPM is a rare breed, combining technical expertise with business acumen and a passion for solving real-world problems with data. They are the translators, bridging the gap between complex data capabilities and strategic business objectives.
Imagine a skilled data analyst like Anna, who's been knee-deep in customer data for years. One day, she has a lightbulb moment and realizes her true calling is to bridge the gap between the tech world and the business realm. She transforms into a DPM, becoming the translator everyone's been waiting for - with a data background, customer and stakeholder empathy, first principles thinking, and ideally, a growth focus.
Now, being a DPM is no walk in the park. It's a juggling act with seven critical responsibilities:
- Setting crystal-clear goals for the data team, keeping them laser-focused.
- Connecting the dots between data initiatives and overarching business strategies.
- Crafting a roadmap for data products that make a difference.
- Ensuring seamless collaboration and alignment across teams.
- Upholding data quality standards, guaranteeing insights you can bank on.
- Quantifying the impact of data products, keeping the purse strings loose by finding ROI.
- Advocating for the resources the data team needs to knock it out of the park.
By fulfilling these responsibilities, DPMs align data initiatives with business goals, architecting requirements for projects that personalize customer interactions, optimize inventory levels, or reduce churn. They are the guardians of data quality, the advocates for resources, and the storytellers who quantify the transformative potential of data-driven decision-making.
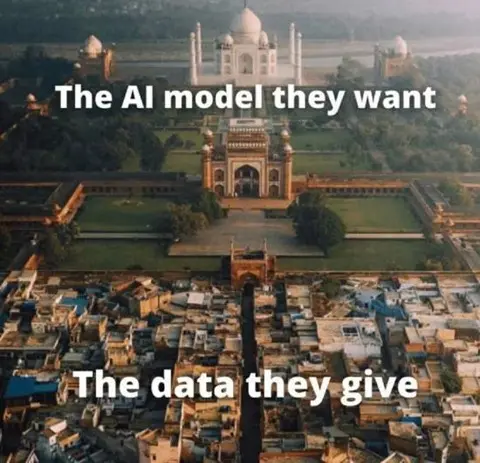
In the age of generative AI, the DPM's role takes on even greater significance. As AI models become increasingly sophisticated, their performance hinges on the quality and relevance of the data they are trained on. DPMs must apply first principles thinking, breaking down problems to their fundamentals and questioning every requirement to ensure that data pipelines are reliable and focused on the intersection of customer and business value.
However, it's crucial to recognize that the typical dynamics between startups and incumbents do not apply in AI as they did in previous technology revolutions like mobile and the Internet. As Jason Cohen astutely points out, ignoring this difference can be perilous for AI startups.
Incumbents are not risk-averse when it comes to AI; they're embracing new technology and uncertain markets, spending historic amounts of money and time. They already have the innovation and the data, while startups struggle to find distribution in over-saturated markets. Top AI and software engineering talent are happy working at incumbents, with above-market rates, exciting projects, healthy budgets, and the ability to impact huge numbers of customers quickly.
Furthermore, there's no such thing as "the AI market" unless you're competing directly with OpenAI. The market for chatbots and SEO tools is the same as before, now with stiffer competition. The typical startup strategies relying on the advantages of David against Goliath are largely untrue in AI.
As we navigate the complexities of the digital age, the rise of the DPM signals a broader shift toward recognizing data as a strategic asset. Much like how product managers revolutionized software development, DPMs are poised to transform data teams from cost centers into revenue-generating powerhouses. By fostering communication, collaboration, and visibility, DPMs will elevate the strategic importance of data teams and unlock the full potential of data-driven innovation.

The future is data-driven, and the DPM is the catalyst that will propel organizations forward. As data's importance continues to grow, so too will the demand for skilled DPMs who can navigate the intersection of technology and business strategy. By embracing the power of generative AI, applying first principles thinking, and developing strategies tailored to the unique dynamics of the AI landscape, DPMs will shape the future of business, turning raw data into actionable insights and transforming the way we work, live, and innovate.
In this era of unprecedented opportunity, the rise of the DPM represents a shift in how organizations approach data. It is a call to recognize the strategic value of data and invest in the talent and infrastructure necessary to harness its full potential. The journey from data to AI is not merely about technology (another hammer); it is about vision (why), strategy (how), and the human touch (who) that connects the dots.
Some great resources to learn more:
- The Value of Data - David Jayatillake
- AI startups require new strategies: this time it's actually different - Jason Cohen
Stay strategic,
Kevin